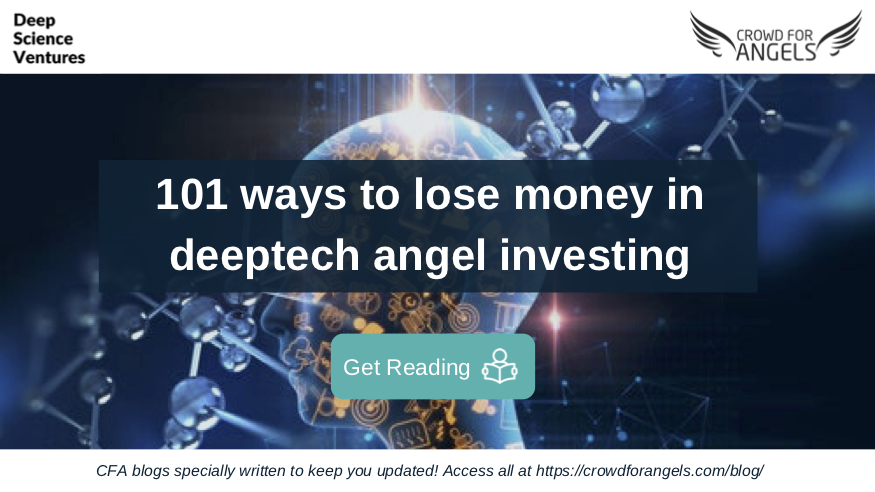
After 10 years of building and backing deeptech companies at one of Europe’s leading universities, in the City and our own fund Deep Science Ventures, one thing is clear. The outcomes can be big and exceed tech outcomes in both scale and speed. But any clues on how to navigate this landscape are largely hidden in hearsay.
Such phrases banded about can include “avoid clean-tech, terrible bust last time”, “hardware is too hard”, “synthetic biology is hot right now” and “ventures around a certain biological target are raising a lot of money recently”. In reality, these are broad terms and often not true. For example, the term ‘cleantech’ represents a hugely diverse set of approaches, there have been some enormous hardware exits and there are more struggling synbio companies than mattress companies.
So it’s critical to pull out the real quantifiable drivers underlying these heuristics to have any chance in deeptech. Moreover, we’ve seen teams completely transform investor feedback just by changing the way they are framed from “microbiome” to “synbio”. This is a sure signal that ‘pattern matching’ is regularly failing to identify commercial or technical fundamentals.
People invest in deeptech for one or both of the following reasons. Firstly, the potential to own a monopoly asset via patent protection – a world-first that gives some breathing room, free from competition to build a uniquely capable company. Or secondly, from the perspective of making a positive impact on the world beyond that which can be achieved with off-the-shelf software alone.
Sadly, as enticing as the novelty is, world class, patented IP alone often adds very little advantage. In fact, if the IP is the principle focus, it can even be a disadvantage, locking the company into a given approach with a false sense of security and revealing your plans to the competition. The second heuristic is ‘an experienced management team’. No doubt having people that know what they’re doing and an existing network helps significantly, but often the world has changed and the same actions this time aren’t as valuable as a first principles perspective on the problem in hand. Here we’ll be exploring how you can think about some of the challenges ahead in tackling problems that require interaction with the bits, atoms and molecules from a market.
Market dynamics, the people and the motivations
Zero risk, zero effort is key: people act on fear, not upside
A potentially sector shifting technology rarely easily fits in the market. A new technology will inevitably fail if it introduces risk vs. existing solutions or makes someone’s life harder. Yet as something new and different it almost certainly will. There are some precedent examples here. Qbotix for example raised $23 million from energy giants to remove the need for expensive motors in large solar panel installations. The solar farm owners didn’t think the change worth the risk and the ultimate winner was the motor company with a $330 million exit. In healthtech, ReVision Optics raised $194 million to develop a far better solution for vision loss but failed principally because the procedure doubled the workload for the clinicians. This is why virtually every primary care diagnostic eventually bites the dust as well. As does a lot of ‘AI for biotech’ software. The user doesn’t want software, they want assets and results. The problem is not primary care diagnostics: it is rather the burden that these diagnostics place on clinicians. This burden on clinicians is quantifiable, as are the factors that more generally tend to lead to category-specific failures.
Avoid unquantifiable binary risk
Another trap is where there is one key thing that needs to be unlocked to enter the market. This is often the case in healthcare. For example, without a rebate code you can’t charge for your service or device and there is very little data to suggest how or when a change might occur. This is what ultimately killed Claritas genomics which had a better solution for pediatric genetic testing. Binary regulatory risk is unavoidable in some sectors but more quantifiable in some than others. Will the EU ever allow genetic modification of food? Who knows. Will the FDA allow a drug with a specific risk profile through? Well there’s a lot of data on previous outcomes to quantify that decision, which is why you will find a lot more biotech investors than synbio agri investors.
Deeptech often has a better IRR and faster time to liquidity vs. tech, but you have to have visibility on the funding journey.
Deeptech is often faster to liquidity and requires less funding than tech, in contrast to hearsay. In biotech specifically, whilst biotech only represents 11% of VC financing it represents 54% of VC backed IPOs and outperforms IRR in tech 27% to 22% with a median exit time of 8 vs. 9 years. The problem is that without thousands of seed-funds as in tech, most companies die simply because they run out of money before they’ve proven the tech and market. Some sectors have active investing ecosystems with very well understood dynamics, such as biotech, whilst others have very few investors due to endless failures of the past such as med-tech, chemicals, materials.
All are served by the major investors once the company is a real company rather than technology development. Grants help here, as do proof of concept projects, but often serve to trap companies in a loop of niche projects with less than ideal partners that is near impossible to escape from. This was the reason behind the demise of BetterHealth, which was spread too thin and misaligned with their partners. The only way to off-set this is to build products, the simplest possible product to get sales. Unfortunately far too many deep-tech starts are research in disguise (RiD?).
Ride shifting market dynamics
The threats are rarely in competing technology but in the diversity of approaches
Look at the problem space rather than the technology space. It’s so easy to be sucked in by something that looks game changing that is often, not even consciously, hiding the real threats. These won’t appear in the company’s 2×2 competitor grid. The ventures in that grid will either live or die for the same reasons, just look at the 200+ amyloid beta antibody trials that have failed so far. How many hours were spent agonising over the minute differences between those in pitch decks when in reality it’s not a competing technology which will win but a completely different approach (more on our approach in Alzhiemers here).
An example of missing the bigger picture is that of much heralded and very well-funded Airware, a drone analytics company. They had clearly made by far the best customer experience for undertaking facility analysis in a very noisy space. But expansion of consumer grade drones made buyers realise that at the top of the market they could buy their own kit and train experts to do it themselves for less, and at the lower end people simply used off the shelf kit, leaving no space for Airware or any of the similar companies.
Trends and supporting technologies shift faster than companies are built
One of the hardest aspects of deeptech investing is marrying the long time horizons of technology development with often rapid shifts in the macro market. Just a year ago clean-tech was a dirty word, now it’s overflowing with investors, from angels to those managing billions in assets, driven by global sentiment that carries real risks to later stage funds. Two years ago trapped ions was the leading option for quantum computing, now it looks almost cute compared to what can be achieved with photonics, driven by completely independent materials research breakthroughs. A startup can’t keep flip flopping between technologies but it can be flexible enough to ride the underlying waves.
Periods of hype and enormous capital injections can cover a lot of mistakes, 23andme is still lauded as a genome driven drug development poster child, yet after 14 years its deals still represent a tiny proportion of the venture funding it has consumed. Likewise several of the well funded west coast synbio companies should ring alarm bells in that they’re starting accelerators and funds to stimulate the market for their services. All you can realistically do here is build as close to application as possible (see scaling below), build for where the market will be when you are product ready, and with a clear funding route at the time. Play the hype if it arrives and gather as much money as you can to live through the troughs in between.
Founder: “All we needed to do was scale”.
Narrator: “it wasn’t”.
There’s a good reason few people invest early in deeptech – even when all of the market aspects covered above are well considered, the translation between discovery and application is rarely an easy path.
This is a particular risk in materials (including semiconductors), chemicals and therapeutic production. The entire ‘cleantech’ biofuels boom and bust was based on assumptions that the economics would be cost comparable to oil at scale, when in reality hundreds of millions was lost due to issues like algae sticking to pipes. Nearly every battery responds very differently at scale. Envia systems quickly lost cycle life once built at scale, whilst Satki3’s deal with Dyson ultimately fell apart as the results weren’t reproducible.
Meanwhile, many approaches simply can’t be manufactured in existing facilities, and whilst it might sound realistic to build a factory for a huge multi-billion pound opportunity there are few investors willing to step in to build factories for things that aren’t yet proven. Ahead of long term deployment costs and risks are just guesses. Take something as fundamentally groundbreaking as immunotherapy which has just demonstrated a step change in cancer survival rates and created enormous wealth for early investors. It was invented over 30 years ago. Making a small number of cells in the lab is one thing, making them at factory scale, or rather convincing anyone to take a bet on this, quite another.
Quantify the risk at first principles and enjoy the ride
Deeptech investing can be hugely rewarding: you can enable life saving therapies, prevent the demise of the planet, launch entirely new computational paradigms and certainly many people have made a lot of money. However, whilst we haven’t listed all 101+ traps here, hopefully it’s clear that there are major buckets of risk that if, paid attention to, can reduce risk, but certainly not all are entirely quantifiable. If you’d like to learn more you’re very welcome to join our deeptech investor community over at dsv.io/investorcommunity
Written by: Guest Author, Mark Hammond (Founding Director at Deep Science Venture)
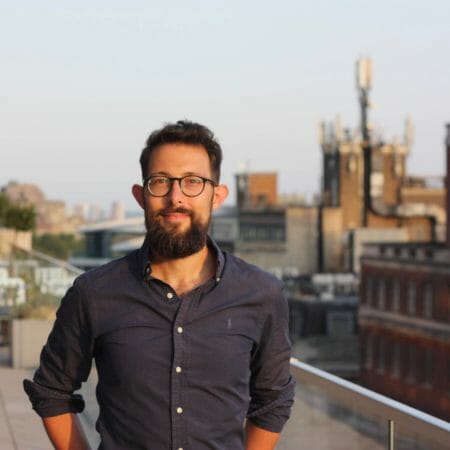
Mark started his career with a PhD in Neuropharmacology and Artificial Intelligence working on better models for the development of epilepsy and neurodegeneration therapeutics, he then worked in cleantech venture before going on to build out Imperial College’s deep-tech start-up accelerator and academic spin-out ecosystem, facilitating several exits to the major tech companies before leaving to start DSV in 2016.
Looking to invest? Or raise funds? Check out Crowd for Angels and explore all opportunities, w: https://crowdforangels.com/